Fully automatic extraction of mitral valve annulus motion parameters on long axis CINE CMR using deep learning
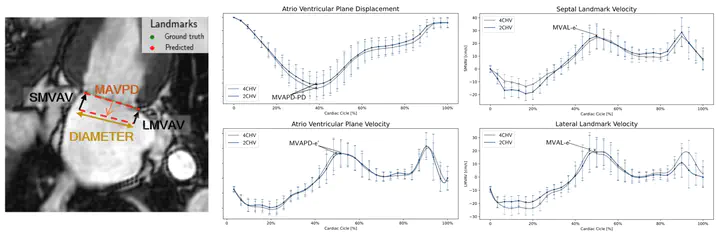
Diastolic dysfunction is an important cause of cardiac insuf- ficiency, defined as a malfunctioning filling of the heart during diastole. The analysis of mitral valve motion is known to be relevant in the diagno- sis of cardiac dysfunction. Cardiac motion parameters can be extracted from Cardiac Magnetic Resonance (CMR) images. However, in clinical setting valve motion modeling usually needs a manual intervention to lo- calize the valvular plane. We propose two chained Convolutional Neural Networks (CNN) for automatic tracking of mitral valve-annulus land- marks on time-resolved 2-chamber and 4-chamber CMR images. The first CNN is trained to detect the region of interest and the second to track the landmarks along the cardiac cycle. The presented deep learn- ing system has high accuracy in terms of temporal landmark tracking and motion assessment. Furthermore, we successfully extracted several motion-related parameters thereby overcoming time-consuming annota- tion and allowing statistical analysis over a large number of datasets.