Myocardial Pathology Segmentation Combining Multi-Sequence Cardiac Magnetic Resonance Images
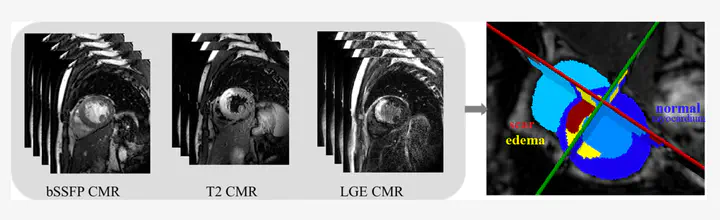
Introduction
Accurate segmentation of myocardial pathological tissue from cardiac magnetic resonance images (CMR), such as scar tissue and edema, f is crutial to the assessment of the severity of myocardial infarction (MI). CMRis the gold standar to provide anatomical and functional information of heart. Specifically, late gadolinium enhancement (LGE) CMR sequence which is used to diagnosis MI, the T2-weighted CMR which resembles ischemic regions, and the balanced- Steady State Free Precession (bSSFP) cine sequence which captures cardiac motions . Combining these multi-sequence CMR data could provide reliable information regarding to the pathological as well as morphological information of the myocardium [1]
Data
The input dataset contains 45 cases of multi-sequence CMR from Myops2020 challenge [1] . Each case refers to a patient with three sequence CMR, i.e., LGE, T2 and bSSFP CMR. All these clinical data have got institutional ethic approval and have been anonymized. The “masks” folder contains 45 cases of multi-sequence CMR, where each mask represents the segmentation map of the the corresponding CMR Slice image:
- Label 1: normal myocardium
- Label 2: edema
- Label 3: scar
- Label 0: Background
The proposed approach involves a two-staged network. First a heatmap-based regression architecture was train to detect a small ROI and locate the myocardium, based on , in order to reduce task complexity. Sequentially a a U-Net based neural network was train to perform multi-modal pathological region segmentation.